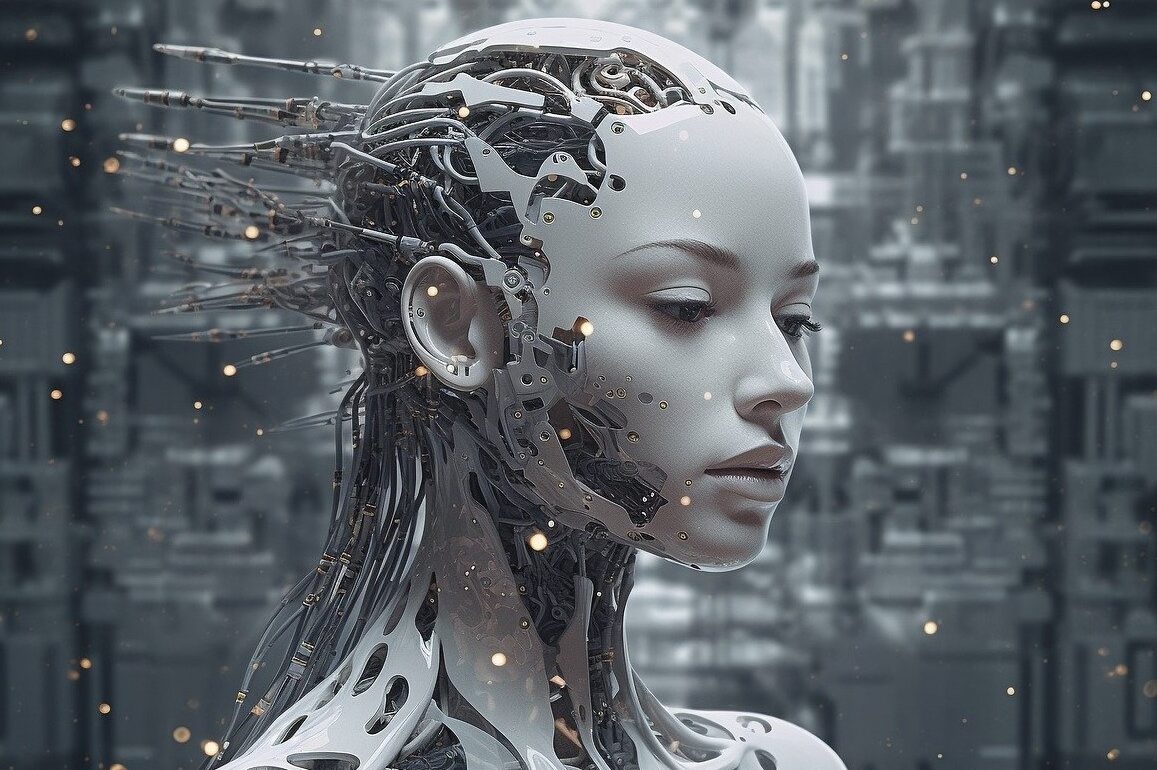
Understanding the historical context and evolution of machine learning not only provides insight into its foundations but also illustrates its progression into the multifaceted technology we see today.
Early Foundations
- Pre-1950s: Theoretical Underpinnings
- Philosophers and mathematicians laid the groundwork for machine learning by exploring logic, human reasoning, and computation.
- Notable Figures:
- Alan Turing: Introduced concepts of computation and the famous Turing Test.
- Norbert Wiener: Pioneered cybernetics, focusing on self-regulating systems.
- 1950s: Birth of AI and ML
- 1950: Turing publishes “Computing Machinery and Intelligence,” which argues that machines can think.
- 1956: The Dartmouth Conference, considered the birthplace of AI, spurred interest in simulating human intelligence.
The Rise of Algorithms
- 1960s: Early Algorithms and Models
- Development of the first neural networks (e.g., Perceptron) by Frank Rosenblatt.
- Introduction of algorithms like the nearest neighbors and decision trees.
- 1970s: Disillusionment and AI Winters
- Initial enthusiasm waned due to limited computational power and unrealistic expectations.
- Funding and interest in AI-related projects decreased significantly.
Resurgence of Interest
- 1980s: Revival through Neural Networks
- Rediscovery of backpropagation techniques powered the neural networks.
- The rise of expert systems led to the commercial application of AI technologies.
- 1990s: Emergence of the Support Vector Machine
- Introduction of Support Vector Machines (SVM) by Vladimir Vapnik, providing a powerful tool for classification tasks.
- Statistical methods gained popularity, leading to a more theory-driven approach to machine learning.
The Big Data Era
- 2000s: Explosion of Data and Computational Power
- The internet boom resulted in unprecedented amounts of data being generated.
- Improvement in hardware, such as GPUs, allowed for more complex computations.
- 2006: Coining of “Deep Learning”
- Geoffrey Hinton and colleagues brought attention to deep learning, marking a pivotal shift in how neural networks could be employed.
- The introduction of frameworks like TensorFlow and PyTorch democratized access to machine learning techniques.
Machine Learning Today
- 2010s: Mainstream Adoption
- Companies like Google, Amazon, and Facebook integrated ML into their products, enhancing user experience and functionality.
- Areas of application expanded: Natural Language Processing (NLP), computer vision, autonomous vehicles, and recommendations systems.
- 2020s: Ethical AI and Explainability
- Dawn of Generative AI (GernAI)
- Growing concerns around ethics, bias, and transparency in ML.
- Researchers emphasize the importance of explainable AI and responsible machine learning practices.
Key Takeaways
- Early theories of computation laid the groundwork for ML.
- The field experienced fluctuations in interest, marked by periods of innovation and AI winters.
- The explosion of data and computational power catalyzed the modern era of machine learning.
- Current trends focus on ethical considerations and the future of AI.